카부캠을 떠날 때가 가까워지는구만~
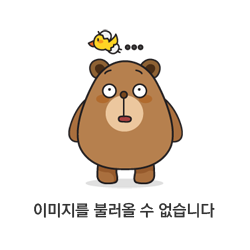
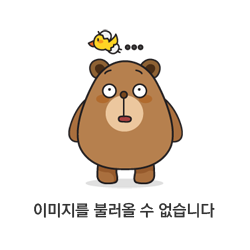
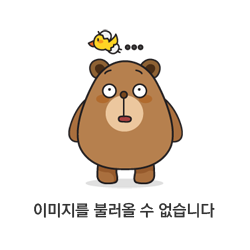
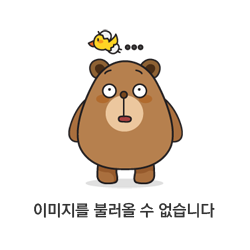
자
- 베이스 모델에 정제한 validation data를 보내서 답변을 얻은 후
- data 안에서 context랑 question, 답변을 answer으로 준비한 프롬프트에 넣어서 gpt 4.o에 쏘고
- 평가용 json을 context, question, answer, evaluation_score(llm 성능 측정 숫자)으로 구성해서 반환
의 계획!
바텀 업 디바이드앤컨커 간다
평가 json 반환기
# 성능 평가 파이프라인
import os
import json
# (키값) context, question, answer, evaluation_score
validate_datas = []
file_path = "fineTuning/data/2.Validation/cutted_validation_data.json"
with open(file_path, "r", encoding="utf-8") as file:
datas = json.load(file)
for data in datas:
validate_data = { "context":"","question":"","answer":"","evaluation_score":-1 }
validate_data["context"] = data["context"]
validate_data["question"] = data["question"]
base_model_answer = # 베이스 모델 응답(data["context"], data["question"])
validate_data["answer"] = base_model_answer
LLM_evaluation_score = # LLM 검증((data["context"], data["question"], base_model_answer)
validate_data["evaluation_score"] = LLM_evaluation_score
validate_datas.append(validate_data)
output_file = "base_model_validation.json"
with open(output_file, "w", encoding="utf-8") as file:
json.dump(validate_datas, file, ensure_ascii=False, indent=4)
LLM 평가 점수 반환기랑 베이스 모델 답변 반환기를 제외하고 짰다
굿?
LLM 평가 점수 반환기
from langchain_openai import ChatOpenAI
from langchain_core.output_parsers import StrOutputParser
from aiModel import llm
import LLMEvaluationPrompt
def get_LLM_evaluation_score(context, question, answer):
formatted_messages = LLMEvaluationPrompt.make_LLM_evaluation_prompt(context, question, answer)
response = llm.AI_model.invoke(formatted_messages)
parser = StrOutputParser()
LLM_evaluation_score = parser.parse(response)
return LLM_evaluation_score.content
4o 모델에게 기존에 만들었던 프롬프트에 매개변수를 넣어서 쏜 후 얻은 답변을 문자열로 파싱해서 반환
베이스 모델 답변 반환기
from transformers import AutoTokenizer, AutoModelForQuestionAnswering
import torch
# 모델과 토크나이저 불러오기 (초기화는 한 번만 수행!!)
model_name = "timpal0l/mdeberta-v3-base-squad2"
tokenizer = AutoTokenizer.from_pretrained(model_name)
model = AutoModelForQuestionAnswering.from_pretrained(model_name)
def get_base_model_answer(context, question):
# 입력 데이터 토큰화
inputs = tokenizer(question, context, return_tensors="pt")
# 모델로부터 예측 결과 받기
outputs = model(**inputs)
answer_start_index = torch.argmax(outputs.start_logits)
answer_end_index = torch.argmax(outputs.end_logits) + 1
# 예측된 답변 토큰을 문자열로 변환
answer = tokenizer.convert_tokens_to_string(
tokenizer.convert_ids_to_tokens(inputs["input_ids"][0][answer_start_index:answer_end_index])
)
return answer
파이토치를 이용해서 베이스 timpal0l/mdeberta-v3-base-squad2 모델에게서 답변을 받는 함수를 만들
통합 실행
만든 두 개의 함수를 결과 json 만들기 py에 연결해 주고,
# 베이스 모델 응답
model_answer = base_model_answer.get_base_model_answer(data["context"], data["question"]) validate_data["answer"] = model_answer
# LLM 검증
LLM_evaluation_score = LLM_evalution_score.get_LLM_evaluation_score(data["context"], data["question"], base_model_answer)
validate_data["evaluation_score"] = LLM_evaluation_score
실행!
하기 전에
중간중간 경과 확인용 콘솔문 넣어야겟다
좋아
싷앻!

변수를 깔끔하게...
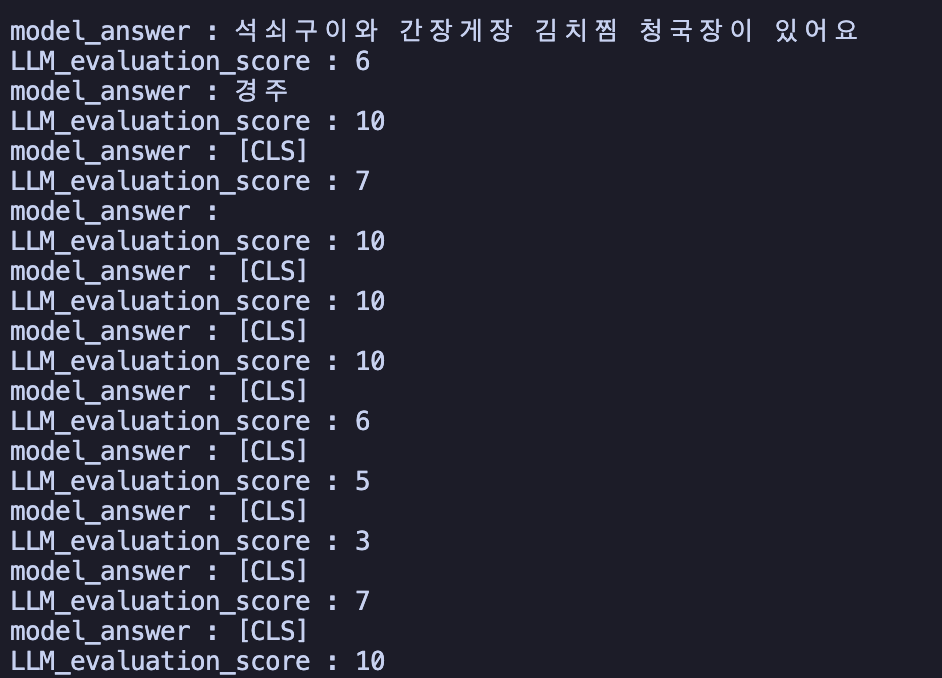
[CLS]는 classification token의 약자로, Transformer 모델(예: BERT, RoBERTa 등)의 입력 시퀀스에서 문장의 시작 부분에 추가되는 특수 토큰
모델이 의도한 답변을 생성하지 못하고 기본적으로 반환된 입력 토큰 중 첫 번째 특수 토큰([CLS])을 결과로 반환했을 가능성
아니 근데
근데 왜 저거에 10점을 주냐 이거야
일단 예외처리..
if model_answer in ["[CLS]", "[SEP]", ""]:
validate_data["evaluation_score"] = 0
print("LLM_evaluation_score : " + 0)
그냥 0점 주자
아냐 그전에 프롬프트 한 번 보고
다행이다!!!
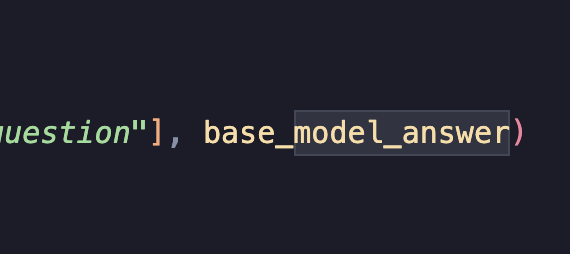
model_answer 넣어야 하는데 함수를!!!

오키...이제 다 점수가 바닥을 기는 문제가 있지만 아무튼...
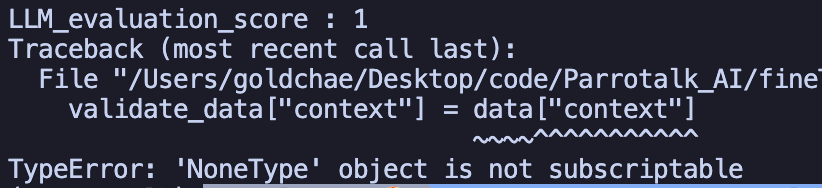
null뭐니...
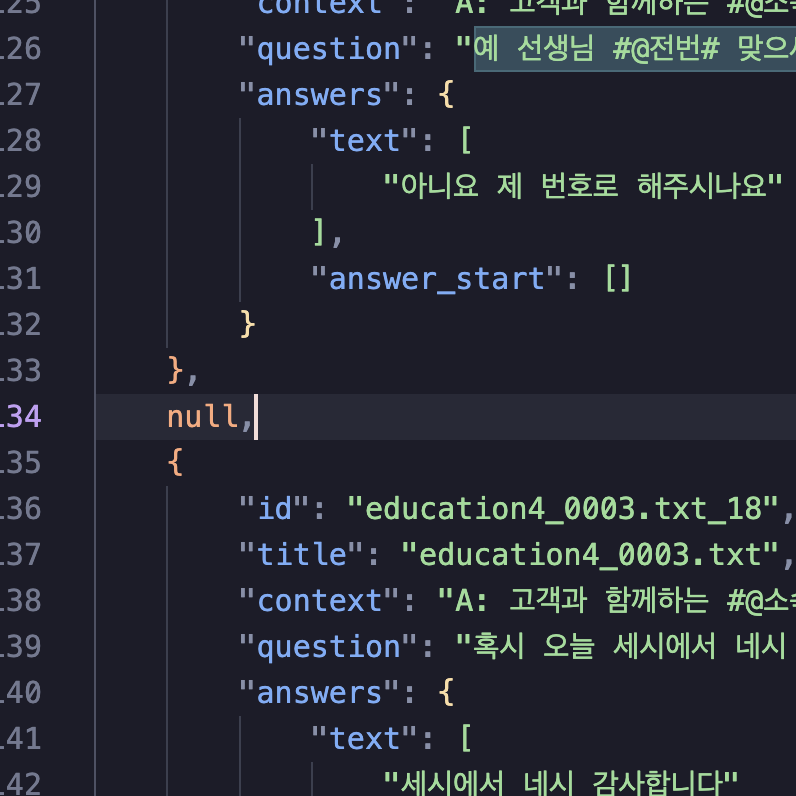
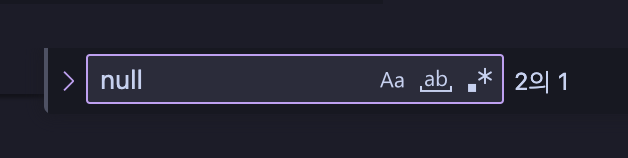
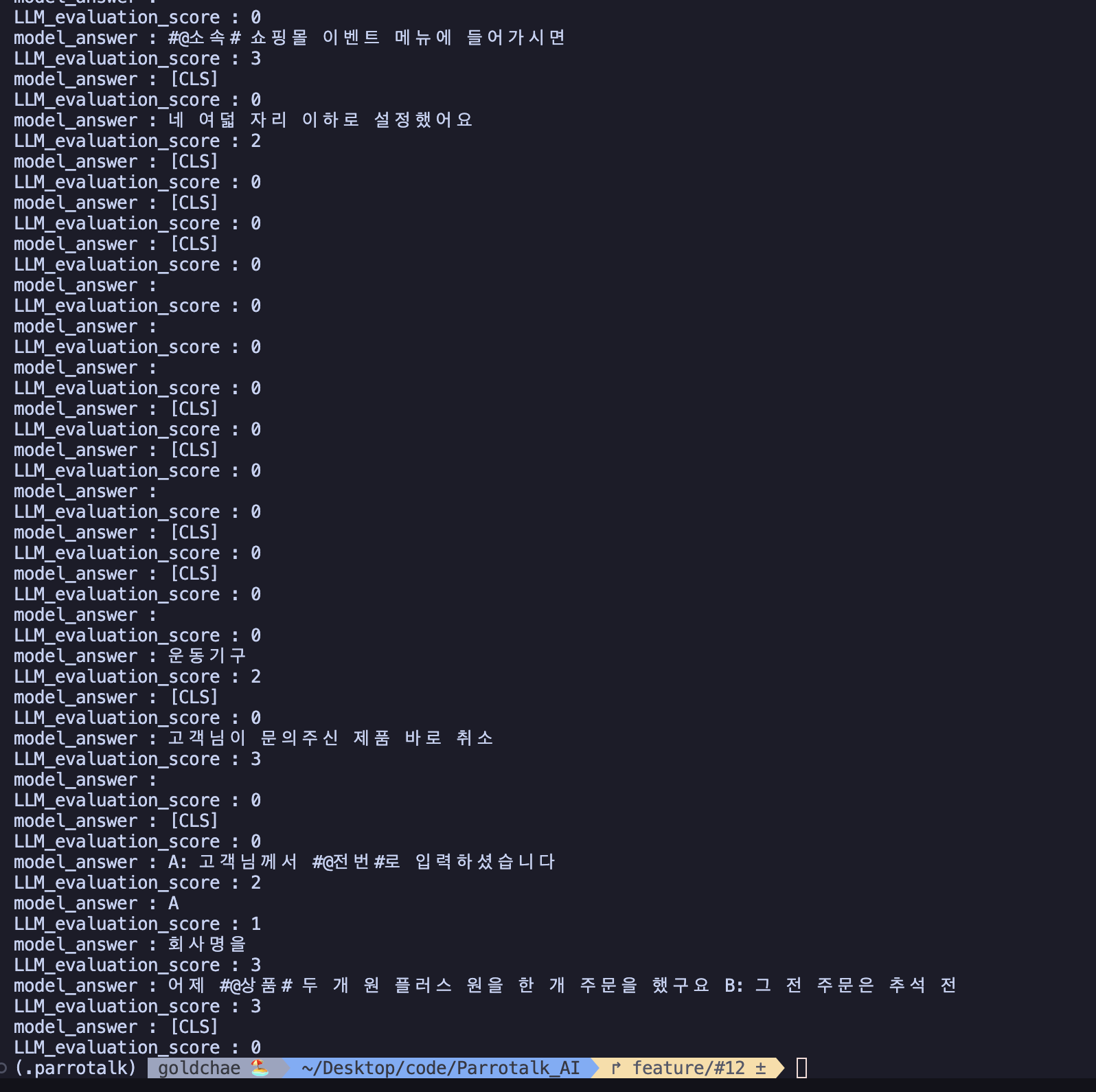
0의 바다에서 간혹 1과 아주 간혹 3이 등장하는군

어쨌든 완성~~~~~
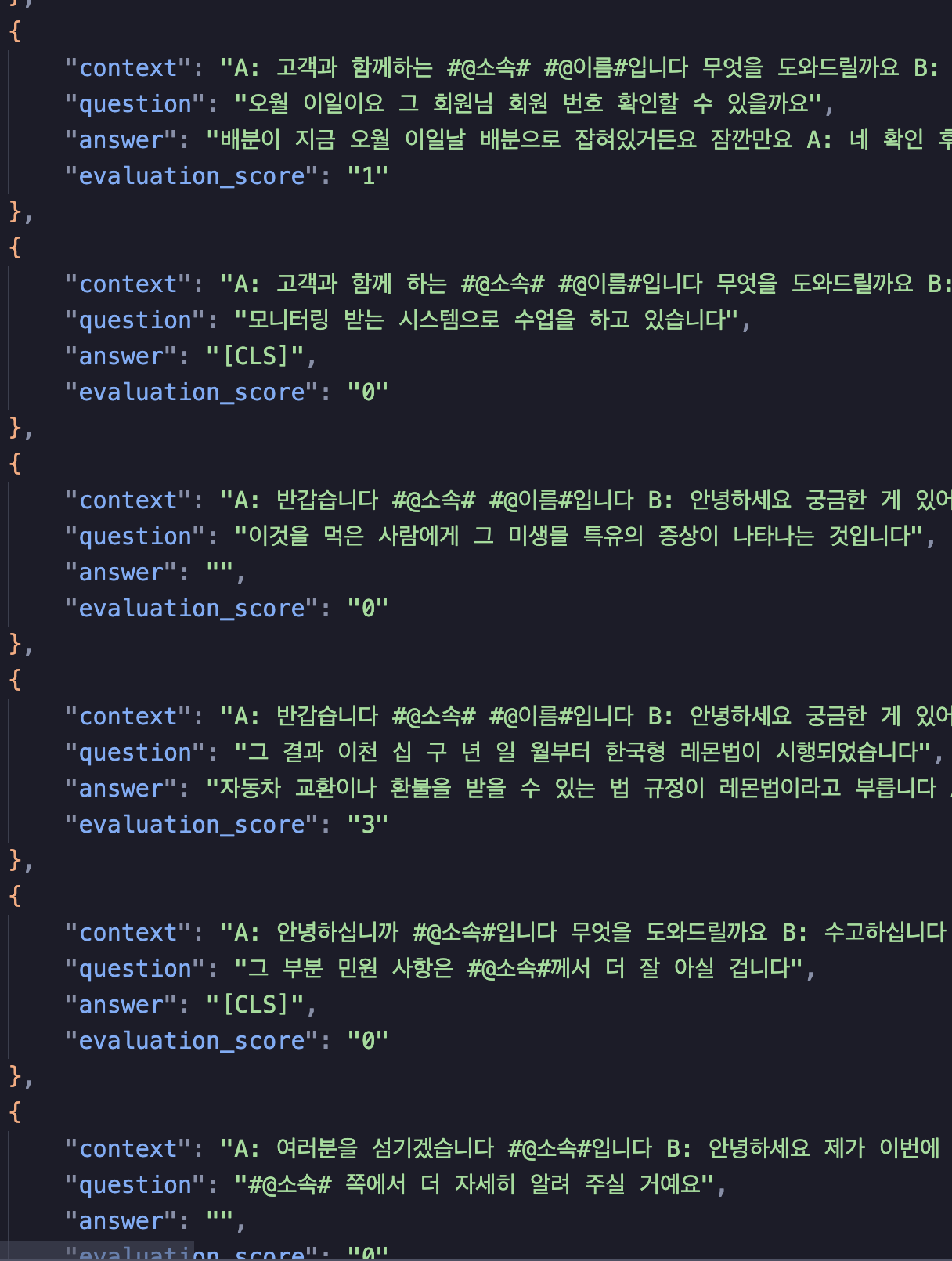
뭔가 전체적으로 끔찍하군
잘 된 것일 수도
성능 향상은 이루어지겠으니..
'Club|Project > 카카오테크 부트캠프 | AI' 카테고리의 다른 글
🦜카부캠 앵무말(Parrotalk) : 성능 통계 (2) | 2024.12.03 |
---|---|
🦜카부캠 앵무말(Parrotalk) : 파인튜닝 모델 성능 측정 (2) | 2024.12.02 |
🦜카부캠 앵무말(Parrotalk) : 검증 데이터 정제 (1) | 2024.12.01 |
🦜카부캠 앵무말(Parrotalk) : 화면 피그마 (2) | 2024.11.28 |
🦜카부캠 앵무말(Parrotalk) : 파인튜닝하기 (1) | 2024.11.25 |